Studies of Color Vision and Light/Matter interactions are 24 centuries old. Plato and Aristotle speculated about color vision. Ancient Greeks reported light-sensitive matter. When certain substances were exposed to light, the substances changed, indicating a chemical reaction. These Light/Matter interactions are the result of photons transferring energy to atoms and molecules. This energy transfer is called quanta catch, or matter’s spectral response to light. When light exposes silver-halide salt, each quanta catch converts a silver ion to a stable silver atom. All Light/Matter interactions take place in the spatial dimensions of angstroms.
Human vision captures information over a solid angle of >140°. It begins by optically imaging light on receptors in the retina. After that it sends the quanta catch information from 100,000,000 receptors to the brain, using only 1,000,000 optic nerves. From neural junctions every receptor sends its light responses to neurons. These neurons make Spatial Comparisons to make visual Appearances.
Traditional photography, movies, and analog videos are records of sensors’ responses to quanta catch. Today, we talk of picture elements, or pixels. Cameras capture light, and record it as digital pixel values. Imaging technologies transmit the array of all those digit values to different media: print, television, and digital displays. The vast majority of imaging technologies perform this task one pixel at a time. Namely the capture, transmission, and presentation to observers works the same as a landline telephone wire connecting the sensor response with the display output. These imaging pipelines, from photon capture to display, are constant for all local image segments. Every segment is processed separately - independent of all the signals from all the other segments.
In 1961, as a Freshman at Harvard, I began a part-time Lab Tech job for Edwin Land, and Nigel Daw of Polaroid in its Vision Research Laboratory. Land’s study of Red and White (Two-Color) Photography made him realize that vision is fundamentally different from photography. Light/Matter interactions are limited to a single pixel’s response to light. Silver halide film responds to individual microscopic scene segments, independent of the rest of the scene. However, Land realized that visual Appearances respond to the spatial content of the entire scene, after the receptors’ responses. In the mid-1960’s Land and I spent time discussing vision’s response to the Natural Scene. One of the themes we discussed was “How human spatial vision worked”. Could understanding human spatial vision lead to better photographs? Land was equally fascinated with both Color challenges: Theory and Practice. How could technology “mimic human vision”? Over the decades that challenge was refined to become: accurately capture light from the entire scene, calculate appearances of all scene segments (using multi-resolution spatial comparisons), and write all appearances on film, now media.
This figure illustrates three stages of photography. The three photos were taken out of the same latticed window in Fox Talbot’s home, Lacock Abbey, Wiltshire, UK. Figure A is a reproduction of Talbot’s 1835 negative photo. It is the oldest surviving silver halide negative image. Figure 1B is a digital rendition of the same scene using three fixed (R,G,B) tone-scale reproduction responses to spectral light. This photo mimics the combined positive and negative responses of Kodacolor and Fujicolor prints. In other words, it mimics the pipeline process that made most color photo prints from 1940 to 2000. Each input pixel determined the value of the output pixel. All pixels used the same pipeline (Tone Scale Function). Figure B is a pixel-by-pixel rendition of the films’ quanta catch.
Three photos of Fox Talbot’s window. A is a reproduction of Talbot’s surviving negative. B illustrates color prints made using 3 (RGB) fixed Tone Scale Curves (eg. Kodacolor). C Digital camera image made with spatial comparisons to compress the scene’s high range of light to fit the print’s low range of reflectances.
Figure C of fox Talbot's window is an icon of photography that mimics human vision. It is a digital photograph using HP’s Digital Flash image processing that incorporated Frankle and McCann’s (1983) Retinex algorithm. The image used spatial comparisons to transform the scene’s high-dynamic-range into a low-dynamic-range image for printing. By emphasizing edges and controlling gradients the algorithm compressed the scene’s range of light to render the Appearances of the bright garden and dim interior.
Vision’s response to the Natural Scene is to build Appearances out of multi-resolution spatial comparisons. These spatial comparisons tend to cancel the effects of optical glare by transforming quanta catch into a new unique rendition of the scene. Optical glare’s scene transformation responds to the distribution of light in the scene. Vision counteracts glare by neural spatial processing that responds to the distribution of light on the retina. (McCann & Rizzi, The Art and Science oh HDR Imaging, 2012). The goal of LM Retinex is to mimic human vision adding vision’s spatial-image processing after technology's receptor quanta. Silver halide photography used fixed tone-scale responses for all scenes. These tone scles converted quata catch into print density. Early digital cameras used the samei process with single pixel pipelines that copied silver halide photography.
Until digital image processing, photography lacked the technology to compare quanta catches from every part of the scene, the way that vision does. Today, digital photography is moving from Tone Scales to Spatial Processes to improve its rendition of all sceines. LM Retinex's goal is to mimic vision's receptor quanta catches after intaocular glare, and neural spatial comparisons interact with the Natural Scene, and other complex images. LM Retinex emphasize that vision’s spatial comparison network vastly expands our ability to do much more than just count quanta.
Rendering of HDR scenes using Spatial Comparisons
Successful HDR Spatial Processes: (A) daVinci painting; (B) van Honthorst’s painting with local normalization; (C) Retinex algorithm;
In Figure A Leonardo da Vinci made Chiaroscuro paintings around 1500 that renders both objects and illumination.
Figure B shows van Honthorst’s 1620 painting of four figures at different distances from a candle, in different levels of illumination. The painter used local maxima to compress the range of the scene. Below the painting is a plot of appearances of 4 pie-shaped gray scale transparencies. The candle and the faces of the four people are local maxima. They darken at a very low rate (Green line). The scenes around them have a very high rate. This recent psychophysical experiment gives quantitative calibration to the Appearances that van Honthorst observed 500 years ago.
Figure C shows “John at Yosemite, 1981” digital print of multi-resolution calculated Appearances on film. The HDR photo was taken in Yosemite Valley. The shade of the tree had 30 times (5 stops) less illumination than the sunlight. Spot photometer readings from John’s white card (in shade) were equal to ColorChecker®’s black square (in sunlight). The sun-shade scene’s dynamic range was 10 stops, or 1,000:1. Color print’s range cannot reproduce this scene. However, color negatives have >1,000: 1 sensitivity range. Figure 8C used color negative film to capture the scene; an Itek scanner to digitize it; and Frankle and McCann’s (1983) multi-resolution Retinex image processing to calculate appearances to reduce the output range to 30:1 to print on film. [See B. LM Retinex Details below]
Successful HDR Spatial Processes: Da) Control; (Db) HP Retinex Camera; (Dc) Apple iPhone X.
Figure D shows three photos of the same scene; (left Da) is a control photo using a fixed RGB tone-scale pipeline for pixels; (Db) is an HP’s Digital Flash implementation of Frankle and McCann’s Retinex algorithm (1983); (Dc) is a iPhone X synthesis of local regions selected from many different exposures. By selecting the image segments with best local rendition and fusing it with other optimal renditions from different images it synthesizes the best overall rendition. All of these techniques of painting, calculating, and firmware processing are different, but they make renditions that human vision accepts as an Appearance record of the entire original scene. These very different technologies all mimic vision’s response to the Natural Scene. They all render the HDR scenes in LDR images. They are all spatial transformations of scene radiances. The most important feature of Figure 8’s processes is they work in all scenes, HDR and LDR. Unlike pixel pipelines, vision and multiresolution spatial comparison algorithms successfully process all scene ranges in all types of scenes. Electronic Imaging has begun to learn what painters did 6 centuries ago.
Electronic Imaging is beginning to mimic our color vision’s
interpretation of Natural Scenes
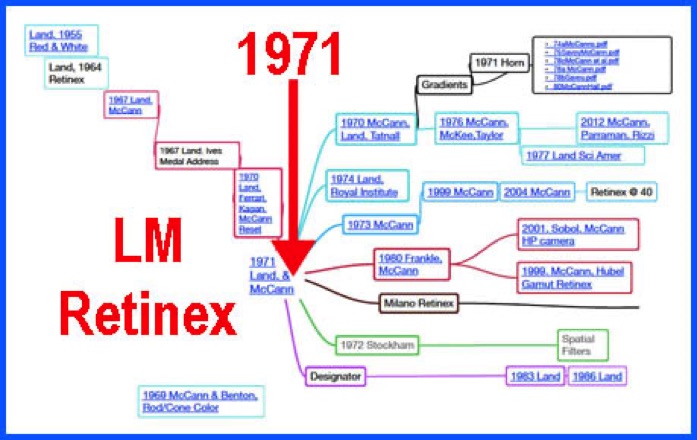
C. Links - All LM Retinex papers and patents in chronological sequence
Land & McCann Retinex
A. Overview - A brief summary of goals, accomplishments, and what it does.
B. Details - Part 1 describes the original LM-Retinex model
Part 2 describes other Retinex Models
C. Links - All LM Retinex papers and patents in chronological sequence
Land & McCann Retinex
A. Overview - A brief summary of goals, accomplishments, and what it does
Land & McCann Retinex
B. LM Retinex Details - Part 1 describes the original LM-Retinex model
The goal of the LM-Retinex algorithm was to mimic the neural spatial image process of human vision. Assume that human vision is a physicists black box. Its input is calibrated scene radiances of all scene segments; its output is the Appearance of all segments. If we assume that human vision has a fixed neural network that processes all scenes using fixed mechanisms, can we predict the behavior of this Appearance network from the accumulated visual responses from many scenes?
The original idea was to make quantitative measurements of all Appearances in many different, difficult scenes. Namely, the scenes in which quanta catch did not predict matches (eg. Color Constancy, Contrast, Gradients). The quantitative values were matches to a color catalog (eg. Munsell Book) with constant surround, in constant, uniform illumination.
Using calibrated radiances (not camera digits) the LM Retinex algorithm calculated the predicted match of all scene segments. The plot of predicted Appearance vs. observer match of each segment was used to tune the image processing parameters. LM Retinex used all the accumulated individual quantitative matches from many scenes to define the algorithmic spatial-image response to all scenes. In order to mimic vision, it is necessary to measure vision's ground-truth response to all scenes. The LM Retinex is the only algorithm whose parameters were selected using the collected ground truth data from many difficult scenes.
Rather than asking an observer if they preferred the look of the algorithm's output image for an individual uncalibrated photograph, LM Retinex was designed to transform scenes’ radiances the way that human vision transforms the spatial content.
Part 1 begins with McCann, Land and Tattnall’s (1970) technique for quantifying Appearances in many scenes, and continues to digital cameras that captures all scene radiances, calculates all appearances, and displays them on media.
Land & McCann Retinex
B. LM Retinex Details - Part 2 describes other Retinex Models
Over the 50 years since the publication of the LM Retinex model of visual Lightness, the term Retinex Model has expanded, and has been transformed. Today, this family of models called “Retinex” include vastly different initial assumptions, different inputs, conflicting goals, and make different output images. It is not possible to rewrite history, so this brief review simply compares and contrasts the family of Retinex properties, assumptions, and results. Some examples, such as Frankle & McCann (1983); Sobol & McCannl (2002); Gamut Mapping Retinex (2002) incorporate the original LM Retinex algorithm, others do not.
This begs the question,”What are the fundamental assumptions of LM-Retinex models?”. They are:
• Model input - calibrated scene radiances of all scene segments
Vision model - calibrated L, M, S cone quanta catch
Camera model - calibrated R, G, B scene radiances
• Independent L, M, S spectral channels that make spatial comparisons to calculate the apparent triplet of L,M,S lightness of all scene segments
• Spatial comparison calculation is tuned using ground-truth observer matches
accumulated over many difficult scenes (Color constant, contrast, gradients)
• LM Retinex goal is to calculate the appearance (matches) of all scene segments
Vision Model - Calculate matching chip in Munsell book
Camera model - Calculate Appearances
Convert HDR scene into LDR Appearances
• Use profile to scale output into display’s color space
• LM Retinex Appearance mimics human vision.
An essntail property of LM Retinex is that it replaces the 18 century notion that vision is able to recognize the surface reflectance of objects by discounting the illumination.
Land wrote the last sentence in Land and McCann (1971) that says:
"...the function of retinex theory is to tell how the eye can ascertain refiectance in a field in which the illumination is unknowable and the refiectance is unknown."
Here Land make the point that in his Color and Black & White Mondrian experiments, observers reported that Apperances correlated with the Mondian papers' reflectances. Spatial vision's challenge is to calculate Appearance. He believed that it is not possible to calculate illuminatuon, or reflectace from all scenes' radiances. ("the illumination is unknowable and the refiectance is unknown).
It is ironic that some of the Family of Retinex algorithms claimed that LM retinex calculated surface reflectance. The important fact is that in Natural Scenes observers' Appearances do not correlate with surface reflectances. (McCann, Parriman & Rizzi, 2014; McCann, 2021). LM Retinex would fail it primary goal of mimicking human vision, if it did calculate reflectance. Vision does not solve that problem. Nevertheless, some of the Retinex family literature makes this claim.
The accumulated obsever matches from many difficult scenes provided abundant evidentce that Appearances are controlled by Edges and Gradient in scene radiance, not the antiquated idea of Reflectances and Illuminations.
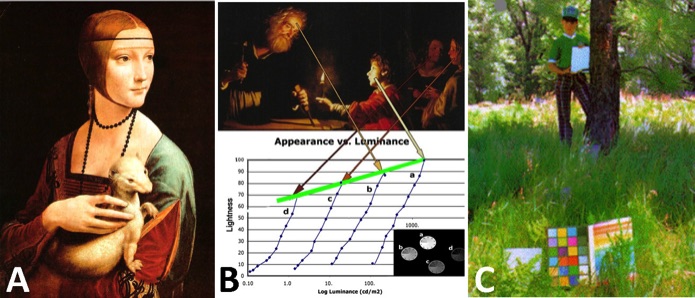
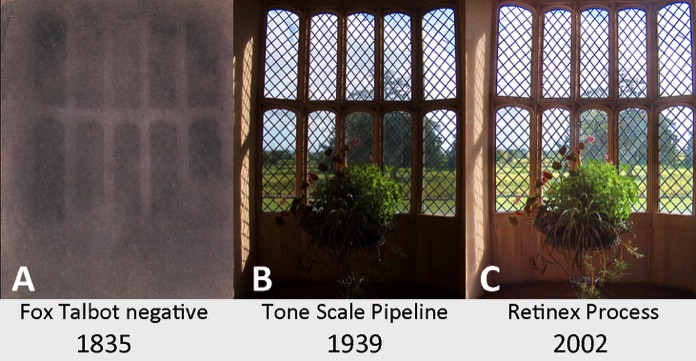
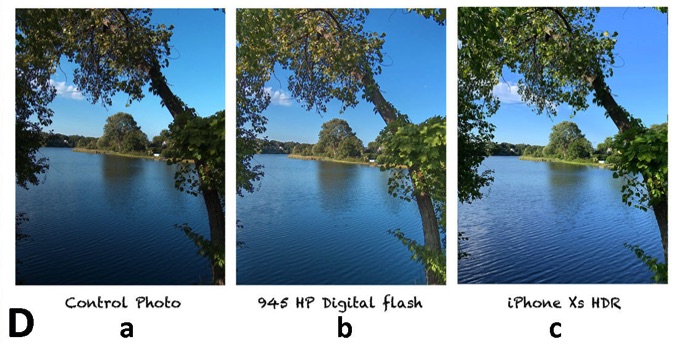